Explore this course:
Apply now for 2025 entry or register your interest to hear about postgraduate study and events at the University of Sheffield.
Statistics with Financial Mathematics
School of Mathematical and Physical Sciences ,
Faculty of Science
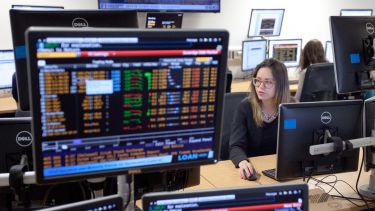
Course description
Our Statistics with Financial Mathematics MSc trains you to apply the probabilistic, statistical and mathematical techniques that are used in the finance industry.
In addition to a variety of statistical techniques, we’ll cover key financial topics such as the Capital Asset Pricing Model, the Black-Scholes option pricing formula and stochastic processes.
You’ll develop a detailed working knowledge of important statistical techniques and concepts, including linear and generalised linear modelling, Bayesian statistics, time series and machine learning.
You’ll learn how to analyse and draw meaningful conclusions from data, and develop your programming skills using the statistical computing software R.
Around one-third of the course is devoted to your dissertation. This may focus on investigating a data set, or a more theoretical or methodological topic. You’ll gain skills to help you stand out in the graduate job market, such as planning and researching a project, data acquisition, problem specification, analysis and reporting your findings.
External clients, such as pharmaceutical companies or sports modelling organisations, often provide dissertation topics. Distance learning students often come with projects designed by their employer.
Recent examples of dissertation topics include:
- Financial modelling with Lévy processes
- Contagion in Financial Networks
Accreditation
Accredited by the Royal Statistical Society
Modules
Core modules:
- Financial Mathematics
-
The discovery of the Capital Asset Pricing Model by William Sharpe in the 1960's and the Black-Scholes option pricing formula a decade later mark the beginning of a very fruitful interaction between mathematics and finance. The latter obtained new powerful analytical tools while the former saw its knowledge applied in new and surprising ways. (A key result used in the derivation of the Black-Scholes formula, Ito's Lemma, was first applied to guide missiles to their targets; hence the title 'rocket science' applied to financial mathematics). This course describes the mathematical ideas behind these developments together with their application in modern finance, and includes a project.
15 credits - The Statistician's Toolkit
-
This is the first of two 'core' modules students studying on statistics MScs. The aim of this module is to prepare statisticians for the workplace, equipping them with essential statistical modelling, computing and professional skills. The module includes the study of linear and generalised linear modelling, and data analysis using the programming language R.
30 credits - Bayesian Statistics and Computational Methods
-
This module introduces the Bayesian approach to statistical inference. The Bayesian method is fundamentally different in philosophy from conventional frequentist/classical inference, and has been the subject of some controversy in the past, but is now widely used. The module also presents various computational methods for implementing both Bayesian and frequentist inference, in situations where obtaining results 'analytically' would be impossible. The methods will be implemented using the programming languages R and Stan, and some programming is taught alongside the theory lectures.
30 credits - Stochastic Processes and Finance
-
Stochastic processes are models that reflect the wide variety of unpredictable ways in which reality behaves. In this course we study several examples of stochastic processes, and analyse the behaviour they exhibit. We apply this knowledge to mathematical finance, in particular to arbitrage free pricing and the Black-Scholes model.
30 credits - Dissertation
-
The dissertation is an extensive study giving the student the opportunity to synthesise theoretical knowledge with practical skills and giving experience of the phases of a relatively large piece of work: planning to a deadline; researching background information; acquisition and validation of data; problem specification; the carrying through of relevant analyses; and reporting, both at length through the dissertation and in summary, through, for example, a poster display. Most dissertations involve the investigation of a data set, entailing both a description of the relevant background and a report on the data analysis.
60 credits
Optional modules:
A student will take 15 credits from this group.
- Machine Learning
-
Machine learning lies at the interface between computer science and statistics. The aims of machine learning are to develop a set of tools for modelling and understanding complex data sets. It is an area developed recently in parallel between statistics and computer science. With the explosion of “Big Data”, statistical machine learning has become important in many fields, such as marketing, finance and business, as well as in science. The module focuses on the problem of training models to learn from training data to classify new examples of data.
15 credits - Time Series
-
This module considers the analysis of data in which the same quantity is observed repeatedly over time (e.g., recordings of the daily maximum temperature in a particular city, measured over months or years). Analysis of such data typically requires specialised methods, which account for the fact that successive observations are likely to be related. Various statistical models for analysing such data will be presented, as well as how to implement them using the programming language R.
15 credits
Year one core modules:
- Financial Mathematics
-
The discovery of the Capital Asset Pricing Model by William Sharpe in the 1960's and the Black-Scholes option pricing formula a decade later mark the beginning of a very fruitful interaction between mathematics and finance. The latter obtained new powerful analytical tools while the former saw its knowledge applied in new and surprising ways. (A key result used in the derivation of the Black-Scholes formula, Ito's Lemma, was first applied to guide missiles to their targets; hence the title 'rocket science' applied to financial mathematics). This course describes the mathematical ideas behind these developments together with their application in modern finance, and includes a project.
15 credits - The Statistician's Toolkit
-
This is the first of two 'core' modules students studying on statistics MScs. The aim of this module is to prepare statisticians for the workplace, equipping them with essential statistical modelling, computing and professional skills. The module includes the study of linear and generalised linear modelling, and data analysis using the programming language R.
30 credits
Year one optional modules:
A student will take 15 credits (one module) from this group.
- Machine Learning
-
Machine learning lies at the interface between computer science and statistics. The aims of machine learning are to develop a set of tools for modelling and understanding complex data sets. It is an area developed recently in parallel between statistics and computer science. With the explosion of “Big Data”, statistical machine learning has become important in many fields, such as marketing, finance and business, as well as in science. The module focuses on the problem of training models to learn from training data to classify new examples of data.
15 credits - Time Series
-
This module considers the analysis of data in which the same quantity is observed repeatedly over time (e.g., recordings of the daily maximum temperature in a particular city, measured over months or years). Analysis of such data typically requires specialised methods, which account for the fact that successive observations are likely to be related. Various statistical models for analysing such data will be presented, as well as how to implement them using the programming language R.
15 credits
Year two:
- Bayesian Statistics and Computational Methods
-
This module introduces the Bayesian approach to statistical inference. The Bayesian method is fundamentally different in philosophy from conventional frequentist/classical inference, and has been the subject of some controversy in the past, but is now widely used. The module also presents various computational methods for implementing both Bayesian and frequentist inference, in situations where obtaining results 'analytically' would be impossible. The methods will be implemented using the programming languages R and Stan, and some programming is taught alongside the theory lectures.
30 credits - Stochastic Processes and Finance
-
Stochastic processes are models that reflect the wide variety of unpredictable ways in which reality behaves. In this course we study several examples of stochastic processes, and analyse the behaviour they exhibit. We apply this knowledge to mathematical finance, in particular to arbitrage free pricing and the Black-Scholes model.
30 credits - Dissertation
-
The dissertation is an extensive study giving the student the opportunity to synthesise theoretical knowledge with practical skills and giving experience of the phases of a relatively large piece of work: planning to a deadline; researching background information; acquisition and validation of data; problem specification; the carrying through of relevant analyses; and reporting, both at length through the dissertation and in summary, through, for example, a poster display. Most dissertations involve the investigation of a data set, entailing both a description of the relevant background and a report on the data analysis.
60 credits
Year one:
- The Statistician's Toolkit
-
This is the first of two 'core' modules students studying on statistics MScs. The aim of this module is to prepare statisticians for the workplace, equipping them with essential statistical modelling, computing and professional skills. The module includes the study of linear and generalised linear modelling, and data analysis using the programming language R.
30 credits - Financial Mathematics
-
The discovery of the Capital Asset Pricing Model by William Sharpe in the 1960's and the Black-Scholes option pricing formula a decade later mark the beginning of a very fruitful interaction between mathematics and finance. The latter obtained new powerful analytical tools while the former saw its knowledge applied in new and surprising ways. (A key result used in the derivation of the Black-Scholes formula, Ito's Lemma, was first applied to guide missiles to their targets; hence the title 'rocket science' applied to financial mathematics). This course describes the mathematical ideas behind these developments together with their application in modern finance, and includes a project.
15 credits
Year two:
- Bayesian Statistics and Computational Methods
-
This module introduces the Bayesian approach to statistical inference. The Bayesian method is fundamentally different in philosophy from conventional frequentist/classical inference, and has been the subject of some controversy in the past, but is now widely used. The module also presents various computational methods for implementing both Bayesian and frequentist inference, in situations where obtaining results 'analytically' would be impossible. The methods will be implemented using the programming languages R and Stan, and some programming is taught alongside the theory lectures.
30 credits - Stochastic Processes and Finance
-
Stochastic processes are models that reflect the wide variety of unpredictable ways in which reality behaves. In this course we study several examples of stochastic processes, and analyse the behaviour they exhibit. We apply this knowledge to mathematical finance, in particular to arbitrage free pricing and the Black-Scholes model.
30 credits
Year three core module:
- Dissertation
-
The dissertation is an extensive study giving the student the opportunity to synthesise theoretical knowledge with practical skills and giving experience of the phases of a relatively large piece of work: planning to a deadline; researching background information; acquisition and validation of data; problem specification; the carrying through of relevant analyses; and reporting, both at length through the dissertation and in summary, through, for example, a poster display. Most dissertations involve the investigation of a data set, entailing both a description of the relevant background and a report on the data analysis.
60 credits
Year three optional modules:
A student will take 15 credits (one module) from this group.
- Time Series
-
This module considers the analysis of data in which the same quantity is observed repeatedly over time (e.g., recordings of the daily maximum temperature in a particular city, measured over months or years). Analysis of such data typically requires specialised methods, which account for the fact that successive observations are likely to be related. Various statistical models for analysing such data will be presented, as well as how to implement them using the programming language R.
15 credits - Machine Learning
-
Machine learning lies at the interface between computer science and statistics. The aims of machine learning are to develop a set of tools for modelling and understanding complex data sets. It is an area developed recently in parallel between statistics and computer science. With the explosion of “Big Data”, statistical machine learning has become important in many fields, such as marketing, finance and business, as well as in science. The module focuses on the problem of training models to learn from training data to classify new examples of data.
15 credits
The content of our courses is reviewed annually to make sure it's up-to-date and relevant. Individual modules are occasionally updated or withdrawn. This is in response to discoveries through our world-leading research; funding changes; professional accreditation requirements; student or employer feedback; outcomes of reviews; and variations in staff or student numbers. In the event of any change we'll consult and inform students in good time and take reasonable steps to minimise disruption.
Open days
An open day gives you the best opportunity to hear first-hand from our current students and staff about our courses.
Open days and campus tours
Duration
- 1 year full-time
- 2-3 years part-time by distance learning
Teaching
You’ll be taught through lectures, tutorials, computing sessions and group work. Most statistics lectures are recorded so you can watch them again later.
You’ll be expected to spend around 35 hours each week on your studies, with 8-12 hours in lectures or computing classes, and the remainder consisting of independent study.
Distance learning option
Our distance learning option is taught online with support via email and an online forum. Distance learners also come to the University for residential weeks. You'll need to be in Sheffield for a few days between late May and early June each year for your exams.
You're expected to spend around 20 hours each week on your studies if you're doing the two-year version of the course, and around 12 to 15 hours each week if you're doing the three-year version.
Assessment
Our assessment methods are designed to support the achievement of learning outcomes and develop your professional skills. This includes ongoing project work for some modules, examinations, coursework and a dissertation.
Regular feedback is also provided, so you can understand your own development throughout the course.
Your career
Our Statistics with Financial Mathematics MSc is great preparation for roles in the finance sector such as banking, actuarial work, pensions and insurance. Employers that have hired our graduates in finance roles include Goldman Sachs, Lloyds, Aviva, AXA, Amazon, BAE Systems, Deloitte, PwC and the NHS.
You’ll cover advanced topics and gain extensive research training, which is also great preparation for a PhD. Sheffield mathematics graduates have secured postgraduate research positions at many of the world's top 100 universities.
This degree satisfies the eligibility criteria for the Royal Statistical Society’s Graduate Statistician award – a stepping-stone to full professional membership of the RSS and Chartered Statistician status.
School
School of Mathematical and Physical Sciences
The School of Mathematical and Physical Sciences is leading the way with groundbreaking research and innovative teaching.
Our mathematicians and statisticians have expertise across pure mathematics, applied mathematics, probability and statistics. We focus on a variety of topics, from the most abstract questions in algebraic geometry and number theory, to the calculations behind infectious disease, black holes and climate change.
In the Research Excellence Framework 2021, 96 per cent of our mathematical sciences research was rated in the highest two categories as world-leading or internationally excellent.
We have strong links with the Society for Industrial and Applied Mathematics, the Institute of Mathematics and its Applications, the European Physical Society, and the International Society on General Relativity and Gravitation. With the support of the London Mathematical Society, we are also an organiser of the Transpennine Topology Triangle, a key focal point for topology research in the UK.
Mathematics and statistics staff have received honours from the Royal Society, the Society for Mathematical Biology and the Royal Statistical Society, who also provide professional accreditation for our statistics courses.
Entry requirements
Minimum 2:1 undergraduate honours degree in a relevant subject with relevant modules.
Subject requirements
We accept degrees in the following subject areas:
- Applied / Financial Mathematics
- Data Science
- Economic Statistics
- Mathematics
- Statistics;
We may consider other related degree subjects
Module requirements
You should have studied at least one module from Area 1 and at least two modules from Area 2:
Area 1: Mathematics
- Complex analysis
- Complex variable function
- Functional analysis
- Measure theory
- Real analysis
- Real variable function
- Stochastic analysis
Area 2: Probability / Statistics
- Applied statistics
- Bayesian statistics
- Computational statistics
- Data mining/analysis
- Econometrics
- Linear models / generalised linear models
- Markov chains/processes
- Medical statistics
- Multivariate statistics / multivariable statistics
- Non-parametric statistics
- Probability theory/modelling
- Programming languages (e.g. R, Python)
- Sampling / survey design
- Statistical analysis/experiment/modelling
- Statistical software/computing
- Stochastic processes/models/modelling
- Time series
English language requirements
IELTS 6.5 (with 6 in each component) or University equivalent.
If you have any questions about entry requirements, please contact the school/department.
Fees and funding
Apply
You can apply now using our Postgraduate Online Application Form. It's a quick and easy process.
Contact
Any supervisors and research areas listed are indicative and may change before the start of the course.
Recognition of professional qualifications: from 1 January 2021, in order to have any UK professional qualifications recognised for work in an EU country across a number of regulated and other professions you need to apply to the host country for recognition. Read information from the UK government and the EU Regulated Professions Database.