Xingjian Zhao
School of Architecture and Landscape
PhD Research Student
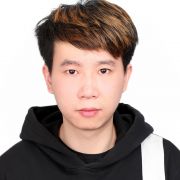
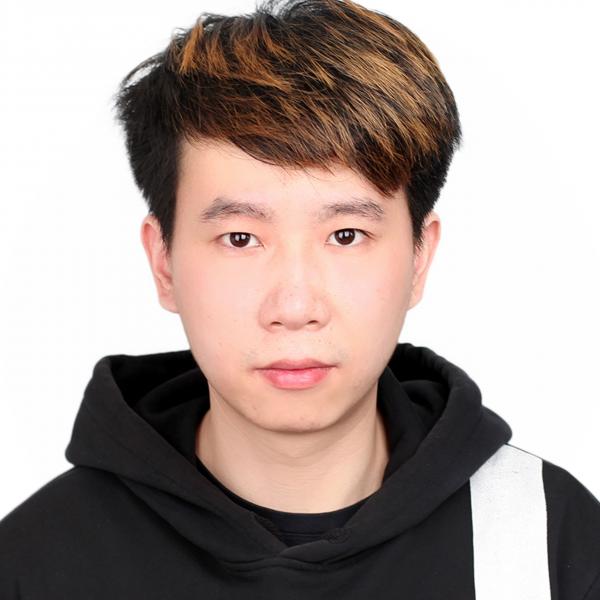
Full contact details
School of Architecture and Landscape
Arts Tower
Western Bank
Sheffield
S10 2TN
- Profile
-
2021 - Present (UK) PhD candidate of Architecture at Sheffield University
2019 - 2020 (UK) MSc in Digital Architecture and Design at Sheffield University
2014 - 2019 (China) BArch in Architecture Design at Chang'an University
I'm interested in the following research topics:
1. Building energy performance evaluation at the early design stage: methods and processes
2. Machine learning based 2D building plan drawing recognition
3. Parametric building performance optimization and design
- Research interests
-
Project title:
An integrated method for building energy performance evaluation at the early design stage using machine learning ——a case study of residential building design in China.
Project outline:
Over the last decades, the global energy consumption increase has led to negative environmental impacts and has been a severe concern that is hindering the development of human society. The building sector is a major energy-intensive sector and accounted for 36% of the final energy consumption and 38% of the total carbon emissions in 2019.
As most building attributes are being decided upon at the early conception design stage, it has great potential to reduce building environmental costs by adjusting the design scheme. In particular, building energy performance simulation is often considered when evaluating building design alternatives during the design process. Due to the frequent design changes, the lack of detailed building information and the uncertainty of design variables in the early design phase, the data-driven methods that use machine learning algorithms are gaining increasing popularity in predicting building energy consumption.
Existing data-driven methods commonly use continuous or discrete numbers to represent the input building design variables to which the accuracy of prediction results is highly sensitive. Among input variables available at the early design stage, building geometrical characteristics and underlying spatial semantics are difficult to quantify yet critical to overall building energy performance. To support building energy performance evaluation at the early design phase, this research focused on the extraction of building geometrical characteristics and reconstructing implicit spatial semantics to develop rapid and accurate building energy consumption prediction based on the Convolutional Neural Network.
Primary supervisor:
Dr Tsung-Hsien WangCo-supervisor:
Dr Chengzhi PengDate started: 01/09/2021
- Research group
-
People, Environments and Performance.