The Role of Meta-Learning for Few-shot Learning
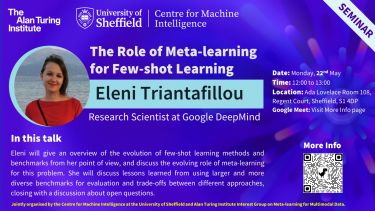
Event details
Description
Abstract: While deep learning has driven impressive progress, one of the toughest remaining challenges is generalization beyond the training distribution. Few-shot learning is an area of research that aims to address this, by striving to build models that can learn new concepts rapidly in a more "human-like" way. While many influential few-shot learning methods were based on meta-learning, recent progress has been made by simpler transfer learning algorithms, and it has been suggested in fact that few-shot learning might be an emergent property of large-scale models. In this talk, I will give an overview of the evolution of few-shot learning methods and benchmarks from my point of view, and discuss the evolving role of meta-learning for this problem. I will discuss lessons learned from using larger and more diverse benchmarks for evaluation and trade-offs between different approaches, closing with a discussion about open questions.
Speaker bio: Eleni Triantafillou is a research scientist at Google DeepMind, based in London UK. She obtained her PhD from the University of Toronto, advised by Professors Richard Zemel and Raquel Urtasun. Her research is centered around creating methods that allow efficient and effective adaptation of deep neural networks to cope with distribution shifts, introduction of new concepts, or removal of outdated or harmful knowledge, falling in the areas of few-shot learning, meta-learning, domain adaptation and machine unlearning.
This seminar is jointly organised by the Centre for Machine Intelligence and Alan Turing Institute Interest Group on Meta-learning for Multimodal Data.
Location
55.378051, -3.435973
When focused, use the arrow keys to pain, and the + and - keys to zoom in/out.