SPandH & Healthcare
Researchers in the Speech and Hearing group are investigating ways of using audio and speech technology to improve the physical and mental wellbeing of people.
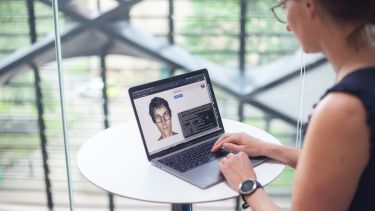
Dr Christensen leads a team investigating different healthcare research projects including the detection and tracking of pathologies in speech and language. As part of a multi-disciplinary team involving expertise in neurology (Dr Dan Blackburn, Prof Markus Reuber), neurophysiology (Prof Annalena Venneri) and linguistics (Dr Traci Walker) they are working to develop a low-cost automatic method for assessing cognitive health. The CognoSpeakTM system (Mirheidari et al, 2019) engages people with memory concerns in a conversation and detects early signs of neurodegenerative disorders like dementia in their speech and language. We are currently working on expanding the system to also track cognitive decline in people with mild cognitive impairment, Parkinson’s Disease and stroke survivors.
Similar speech analytics methods are used by the team to work on automatic methods for the tracking of depression and anxiety in spontaneous, prompted and interactional speech. This includes working with psychologists and psychotherapists to develop approaches for tracking therapist and client interactions in psychotherapy sessions and use this to improve the impact of the intervention.
People with speech pathologies and impairments struggle to use the ever more ubiquitous speech-enabled interfaces on e.g. mobile phones and home-automatic systems as such systems are optimised for typical voices. Christensen is investigating ways of breaking down this barrier and improving the performance of speech recognition and communication aids for people with disordered (dysarthric) speech.
Acoustic analysis is also the focus of Prof Guy Brown and Dr Ning Ma work on the use of deep learning methods to better understand and treat sleep-disordered breathing. Snoring and obstructive sleep apnoea (OSA) are the main forms of sleep-disordered breathing (SDB), which result from the partial and complete collapse of the upper airway during sleep, respectively. OSA has been associated with neurocognitive, cardiovascular and metabolic diseases. The gold standard to diagnose SDB is the polysomnography (PSG) test. However, it is expensive, time consuming, and obtrusive. For this reason, the acoustic analysis of breathing sounds during sleep has been proposed as an alternative to PSG, since it can be applied in an inexpensive and unobtrusive way. The team has shown promising results for their automatic at-home monitoring of sleep disorders using both standard smartphones and newly developed bespoke recording devices.
Snoring and obstructive sleep apnoea (OSA) are the main forms of sleep-disordered breathing (SDB), which result from the partial and complete collapse of the upper airway during sleep, respectively. OSA has been associated with neurocognitive, cardiovascular and metabolic diseases. The gold standard to diagnose SDB is the polysomnography (PSG) test. However, it is expensive, time consuming, and obtrusive. For this reason, the acoustic analysis of breathing sounds during sleep has been proposed as an alternative to PSG, since it can be applied in an inexpensive and unobtrusive way. In this project deep learning is being leveraged to develop tools to potentially screen for SDB such as novel feature representations to classify breathing events in sleep audio recordings (https://ieeexplore.ieee.org/document/8683099, Figure below), a snorer diarisation system that allows the analysis of breathing sounds from two subjects in the same session using single channel sleep audio recordings (paper accepted in ICASSP 2020), and predicting the presence of OSA events in sleep audio recordings by analysing their temporal pattern (abstract accepted in SLEEP 2020).