Professor Andrew Mills
MEng, CEng
School of Electrical and Electronic Engineering
Professor of Innovation in Aerospace Systems Monitoring and Control
Deputy Director Rolls Royce UTC
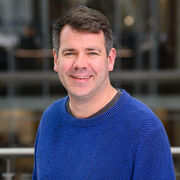
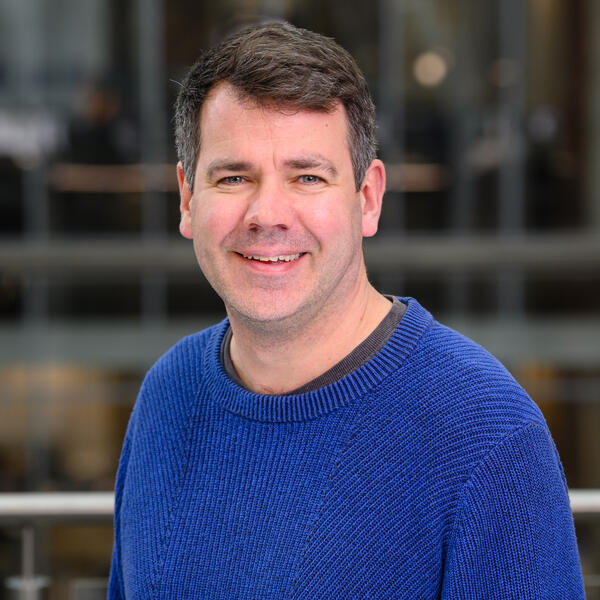
+44 114 222 5634
Full contact details
School of Electrical and Electronic Engineering
Amy Johnson Building
Portobello Street
Sheffield
S1 3JD
- Profile
-
I made an early career decision to follow a path of applied research in Aerospace and return to Sheffield, my undergraduate University, to take a position within the Control Systems Department at Sheffield University. Since then I have worked with industry, particularly Rolls-Royce, to develop sensing and algorithmic solutions to health monitoring and control problems. I have been fortunate to be part of exceptional teams developing fleet monitoring algorithms, vision-based sensing systems, thermal system control laws, and have developed and run various electronic embedded systems in the unforgiving gas turbine environment. I am currently Deputy Director of the Control, Monitoring and Systems University Technology Centre in the School of Electrical and Electronic Engineering at the University of Sheffield in the UK. Here, I shape and co-ordinate a portfolio of industry and research council funded research, PhD projects and MSc projects aimed at delivering high quality academic and industrially exploitable research output.
- Research interests
-
- Equipment health management (EHM) system design,
- Prognostic and diagnostic algorithms,
- Integration of monitoring with control function for through-life adaptable machines.
- Integration of vision sensing and acquisition technologies into systems
- Delivering technology demonstrator programmes with Industrial partners
- Research group
-
Dynamical Systems and Systems Engineering
- Grants
-
- ASUR “Autonomous Multi-criteria Decision Making”, 2015.
- TSB HITEA II, “Advanced Intelligent EHM” (PI), 2014–2017.
- Rolls-Royce, “Control & Systems UTC” (CI), 2013–2015.
- QinetiQ, “Applied Prognostics” (PI), 2013-2015.
- EPSRC, “Through-life Service Feasibility grant” (PI), 2013–2014.
- Rolls-Royce, “Wireless Sensing for Monitoring” (PI), 2013–2015.
- TSB, “SILOET II WP.1.6 – Holistic Control & Health Monitoring” (CI), 2013–2015.
- Rolls-Royce, “Wireless Sensing in Extreme Marine Environment” (CI), 2013–2013.
- Rolls-Royce, “Autonomous Intelligence for Civil UAS” (PI), February 2012–February 2013.
- Teaching activities
-
- AER204 - Embedded Programming
- Professional activities and memberships
-
- Member IMechE / IET / RAeS Joint Propulsion Committee
- Invited Panellist for Prognostics and Health Management conferences
- Reviewer, various Aerospace conferences and Journals
- Lecturer for Industrial Gas Turbine Controls Course in:
- Gas Turbine Control Laws
- Gas Turbine Software Architecture
- Fault Tolerance and Control
- Health Care Monitoring
- System Identification.