Economic Evaluation
Since the School of Health and Related Research's inception in 1994 health economists and mathematical modellers have been working together to produce cost effectiveness analyses for local and national health care decision making bodies.
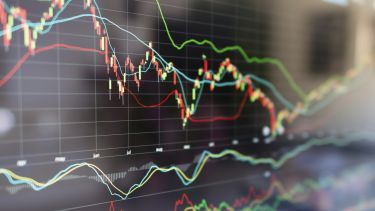
Within our economic evaluation portfolio we undertake both prospective evaluations alongside trials and model-based evaluations based on existing evidence. We are involved in over 50 economic evaluations every year, ranging from small study evaluations through to programmes of research and randomised controlled trials lasting several years. In addition to these current evaluations, we are world-leaders in the development of cutting-edge research methods that are shaping the way that evaluations will be undertaken in the future.
Evaluations alongside prospective studies
The economists within ScHARR work with colleagues and collaborate with other universities to assess the cost-effectiveness of interventions that are being evaluated by prospective evaluations. These studies may be randomised controlled trials, other controlled evaluations, interrupted time-series or single-arm studies. Much of this work is concentrated around ScHARR’s CTRU, CURE and MCRU.
Model-based evaluations
The modellers within ScHARR work in tandem with clinicians, information scientists, reviewers and statisticians to assess cost-effectiveness using existing evidence. This work is principally undertaken as stand-along studies, but can also take place in tandem with the prospective evaluations described previously. Much of this work is concentrated around ScHARR TAG, SARG, NICE DSU and EEPRU.
Alongside the large volume of applied work, members of Health Economics and Decision Science (HEDS) have produced a steady stream of papers on the role of modelling, the methods of modelling and quality assurance in modelling.
HEDS Discussion Papers
This series is intended to promote discussion papers in progress. The Views expressed are those of the authors, and therefore should not be quoted without their permission. However, comments are welcome and we ask that they be sent directly to the corresponding author.
Analysis of uncertainty in cost-effectiveness modelling
HEDS has been increasingly active in research on the analysis of uncertainty in cost-effectiveness modelling. Recent relevant publications are listed below.
Profs. John Brazier and Ron Akehurst were members of the Working Party that produced the most recent NICE Guide to the Methods of Technology Appraisal – a document which mandated probabilistic sensitivity analysis in cost-effectiveness analyses submitted to the NICE Appraisal Programme.
Relevant publications:
- O'Hagan, A., Stevenson, M. and Madan, J. (2005). Monte Carlo probabilistic sensitivity analysis for patient-level simulation models. Research Report No. 561/05, Department of Probability and Statistics, University of Sheffield. Submitted to Health Economics.
- Brennan, A. and Kharroubi, S. A. (2005). Efficient Computation of Partial Expected Value of Sample Information Using Bayesian Approximation. Research Report No. 560/05, Department of Probability and Statistics, University of Sheffield. Submitted to Journal of Health Economics.
- Brennan, A., Kharroubi, S. A., Chilcott, J. and O'Hagan, A. (2005). A two-level Monte Carlo approach to calculating expected value of perfect information:- Resolution of the uncertainty in methods. Submitted to Medical Decision Making.
- Claxton K. Sculpher M. McCabe C. Briggs A. Buxton M. Brazier J. Akehurst R. O’Hagan A. Probabilistic sensitivity analysis for NICE Technology Assessment: Not an Optional Extra. Health Economics 2005;14(4):339-348
- O´Hagan A, McCabe C, Akehurst R, Brennan A, Briggs A, Claxton K, Fenwick E, Fryback D, Sculpher M, Spiegelhalter D, and Willan A. (2005). Incorporation of uncertainty in health economic modelling studies. PharmacoEconomics 23 (6): 529-536
- Tappenden P, Chilcott JB, Eggington S, Oakley J, McCabe C, Methods for expected value of information analysis in complex health economic models: development on the health economic of interferon-beta and glatiramer acetate for multiple sclerosis. Health Technology Assessment 2004;8(27)
Bayesian evidence synthesis
Evidence synthesis involves the development of techniques to combine multiple sources of quantitative evidence. In health technology assessment, meta-analysis is a well-established body of techniques for combining evidence from high-quality trials.
Recently, a number of researchers have been developing methods, grounded in Bayesian statistical theory, to complement and enhance conventional meta-analysis. These methods provide ways of tackling many of the challenges that arise in evidence synthesis, such as heterogeneity, indirect comparisons and baseline risk effects. By explicitly including study quality in the synthesis, these methods are able to draw on a broad evidence base to support decision-making.
Bayesian evidence synthesis is an area of growing interest for HEDS. Current work in this area includes:
- An investigation of modelling methods for integrating routine and trial data in the evaluation of cancer screening programmes (this work is being carried out by Jason Madan for his PhD, funded by a Researcher Development Award from the Department of Health).
- A meta-regression of treatments for Rheumatoid Arthritis (with Dr. Richard Nixon of the MRC Biostatistics Unit).
HEDS also has strong research interests in Expected Value of Information analysis, and Expert Elicitation (BEEP), both of which are related to Bayesian evidence synthesis.
Treatment switching and causal inference in economic evaluation
In randomised controlled trials of new cancer treatments, patients randomised to the control group often switch onto the experimental treatment during trial follow-up, usually after disease progression. But to inform policy, we need to know what would have happened if no control group patients had access to the experimental treatment.
Several statistical methods exist that can be used to adjust for treatment switching to estimate counterfactual outcomes. These methods originated in the causal inference literature. Each method makes important assumptions and will not always be appropriate. Dr Nick Latimer has completed NIHR Doctoral and Post-doctoral Fellowships on this topic, and is currently working on a Senior Fellowship funded by Yorkshire Cancer Research building on this work. He has developed methods, investigated their performance, and written methodological guidance for decision-makers in this area. He teaches on this topic on the Further Statistical Methods for Health Economic Analysis module on the Health Economics and Decision Modelling MSc and together with colleagues in ScHARR has undertaken several applied adjustment analyses for pharmaceutical companies.
Adjustment methods originated in the causal inference literature and were designed primarily to permit the estimation of comparative effectiveness in observational (or real world) data. Investigating the use of these methods for estimating the real world comparative effectiveness of cancer treatments used in the NHS using cancer registry datasets forms part of Dr Latimer’s current research.
Identifying evidence to inform the development of decision-analytic models
Funding: Department of Health Personal Award Scheme
Award Holder: Suzy Paisley
Dates: 2003-2008 (part-time)
Aim
To develop a systematic approach to searching for evidence to inform the development of decision-analytic models.
Background
A key element of systematic reviews and decision-analytic modelling is the process of identifying appropriate evidence in order to reduce bias and uncertainty in the assessment of effectiveness.
Methodological standards for undertaking systematic reviews provide guidance, underpinned by empirical research, on how to search for evidence. Whilst the approach to searching to inform models should be very different from that for systematic reviews, equivalent evidence-based search guidance has not been developed.
Existing good practice guidelines for modelling and for health technology assessment (HTA) stress the importance of incorporating appropriate data. However, guidance on the actual process of data identification is limited to only a few guidelines. Moreover, where this guidance is made explicit, it is brief to the point of being inoperable.
The development of effective search standards for decision-analytic models would bridge a gap in the current methodological guidance. As a consequence the rigour and transparency of assessments used in policy decision-making would be improved.
The aim of this project is to define the requirements of a systematic approach which would allow the planning, execution, management and recording of searches to support decision-analytic models.
Methods
Phase one: Understanding search processes
Participant observation of a heath technology assessment project. The aims of phase one are to assess how and what evidence is used in the model development process and to establish how the model development process might impact on the approach to searching.
Phase two: Developing the search approach
Development of a systematic approach to searching based on the findings of phase one. The process of development includes consideration of how the effectiveness of the search approach should be evaluated. The feasibility of the systematic search approach is evaluated in a second health technology assessment project.
Phase three: Evaluating the search approach
Where phase two focuses on evaluation in terms of process or workability, evaluation of the search approach in phase three focuses on outcome measures including sensitivity, efficiency and reproducibility.
Further information
A presentation on the background to this project was given at two day meeting of the Consensus Working Group on the Use of Evidence in Economic Decision Models. The meeting was funded by the MRC HSRC Workshop Awards Scheme
For further information on this project please contact the award holder, Suzy Paisley.
VTE in Pregnancy
Estimating the value of future research to improve guidelines on thromboprophylaxis for women during pregnancy and after delivery.
Research question
What is the value of undertaking a study to determine the clinical and cost-effectiveness of risk stratification tools for the prediction of venous thromboembolism (VTE) and appropriate provision of thromboprophylaxis for women in pregnancy and after delivery?
Background
Pharmacological prophylaxis to prevent VTE is currently recommended for women who are deemed to be at high risk of VTE during pregnancy or in the 6 weeks after delivery. The decision to provide prophylaxis involves weighing the benefits, harms and costs, which will vary according to the individual’s VTE risk. It is unclear whether the current risk stratification approach could be improved by further research.
Aims and objectives
The aim of the research project is to determine whether further primary research is worthwhile to inform NHS practice on the use of risk stratification tools for the prediction of VTE and appropriate provision of thromboprophylaxis for women in pregnancy and in the puerperium. The specific objectives will be;
1) To estimate the expected costs, health benefits (quality-adjusted life-years) and incremental net monetary benefit of providing thromboprophylaxis using current and alternative risk stratification tools and to quantify the uncertainty around those estimates, given current evidence
2) To determine which factors are the most important drivers of uncertainty when trying to determine the optimal risk-based thromboprophylaxis strategy in this population
3) To identify one or more potential future studies to gather additional evidence that would reduce the current decision uncertainty, whilst being feasible and acceptable to patients and clinicians.
4) To evaluate the value of the potential future research studies in terms of the net health benefits to patients and the cost of the research.
Methods
A decision analytic model will be developed which will be informed by reviews of the existing literature. This model will be used to determine which factors are the most important drivers of uncertainty using expected value of perfect information analysis. Qualitative research, in the form of workshops with patients and a survey of clinicians, will then be undertaken to identify one or more potential future studies that could be conducted to gather additional evidence to reduce the current decision uncertainty. Expected value of sample information analysis will then be used to evaluate the value of the potential future research studies in terms of the net health benefits to patients and the cost of the research.
Timelines for delivery
The project will take place over 15 months with cost-effectiveness modelling (including rapid reviews of parameters) and expected value of perfect information analysis in months 1 to 7, qualitative work to inform potential study designs in months 8 to 10, expected value of sample information analysis in months 11 to 12 and write up and dissemination in months 13 to 15.
Anticipated impact and dissemination
We anticipate that this project will inform the funding of future research studies and may also inform clinical guideline updates. We will share our findings and research recommendations with UK research funders and guideline developers. We will also disseminate professional and plain language summaries to relevant clinical, public and patient representative organisations so they are able to make an evidence-based case for further research or changes to current clinical practice.