Research
The Department of Computer Science is committed to advancing the theoretical foundations of computer science and to achieving impact through close collaboration with end users.
Speech and Language
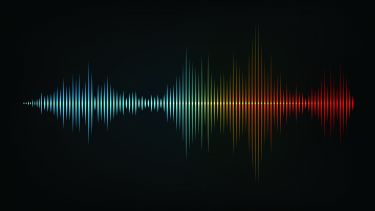
A major challenge for spoken language technology (SLT) is to develop conversational agents that can engage in human-like dialogues: this requires a deep understanding of spoken language behaviour and robustness to the presence of multiple talkers. Explainability, trustworthiness and elimination of bias in SLT systems are also fundamental concerns.
Discover more
Healthcare Technologies
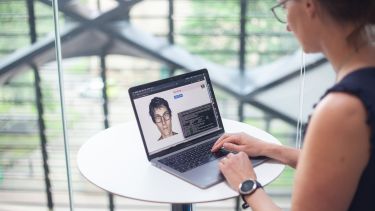
We focus on computational models as tools to identify causal relationships in disease mechanisms. Key challenges include maximising the information gained from images and measurements, developing personalised models to improve diagnosis and target therapy, monitoring disease progression via data analytics, and translating these tools into routine use.
Discover more
Bioinspired Machine Intelligence
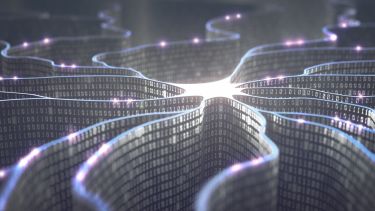
We aim to design energy-efficient algorithms that learn from fewer data, and yield solutions that are interpretable and explainable. We tackle these challenges through fundamental research mainly inspired by biological organisms.
Discover more
Dependable and Secure Systems
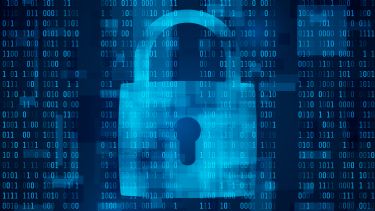
Research is driven by the challenge of developing complex systems that are demonstrably dependable and secure. We address the underlying problems by developing systematic, automated techniques that are grounded in sound theory.
Discover more
We play a central role in the University research environment, providing leadership for four interdisciplinary centres and three flagship institutes.
Research centres
Sheffield Robotics brings together researchers from across disciplinary boundaries to drive forward the understanding, development, and integration of robots and automation.
Insigneo is Europe’s largest research institute dedicated to the development, validation and use of in silico medicine technologies. It aims to transform healthcare by performing cutting edge research in areas of fundamental and applied biomedical modelling, imaging and informatics.
Centre for Assistive Technology and Connected Healthcare (CATCH) is a translational research centre focused on the research, development, implementation and evaluation of new technologies that enable people to live well and age well.
Sheffield Institute for Translational Neuroscience (SITraN) is a world leading centre for research into Motor Neurone Disease, Alzheimer’s and Parkinson’s Disease. It aims to improve the lives of patients with neurodegenerative disorders by conducting basic neuroscience research, developing novel therapies and undertaking clinical trials.
Flagship institutes
Neuroscience Institute
Brings together leading experts in medicine, science and engineering to better understand the nervous system and tackle the biggest challenges in neuroscience.
Healthy Lifespan institute
Over 130 world-class researchers have come together to tackle the global epidemic of multimorbidity to help everyone live healthier, independent lives for longer and reduce the cost of care.
Sustainable food institute
Innovative research draws on the fields of science, engineering, social sciences and arts and humanities to find dynamic solutions to the challenges of food security and sustainability.